The study of the diverse concepts that make up a data set has received significant interest recently because of growing awareness and concerns about data usage.
For the purpose of this article, I like to share some thoughts on data definitions and concepts, including
- values,
- classes, and
- repositories.
Data concepts and definitions
In everyday use, the meaning of "data" varies significantly depending on the situation. Most dictionaries describe “data” as representations of facts about something.
Defining data as facts, however, is faulty thinking.
Data is neutral, neither fact nor fiction. Contemporary interpretation includes data within objective and subjective realms, encompassing all data irrespective of their nature.
Objective data is collected through observations or measurements that are independent of biases. It is this that makes observers agree on what is “given” serving as a basis for shared reflection. It is quantitative in nature and same from multiple sources, provable by means of a test. Examples include sensors generated data, transaction data, census data, and experimental data.
Subjective data is collected by interactions like talking, sharing, explaining, tweets, etc. It is that used to assess the subject’s perspective, feeling, belief, and desire. It is qualitative in nature and varies from one person to another. Examples include questionnaires, interviews, blogs, and editorials.
Another way of looking at “data” is the underlying base. Data with an apparent basis in the physical world is referred to as “Substantial Data” whereas data existing only as an idea or opinion or feeling is classified as “Abstract Data”
Value
In 2017, The Economist published an article entitled, "The world's most valuable resource is no longer oil, but data". While the headline is clear-cut, comparing and measuring data in the same manner as a tangible object is a common mistake in business circles. Some of the inherent data characteristics that distinguish it from a physical entity are:
- firstly, data is an intangible asset with no intrinsic value,
- it acquires value and appreciates in value the more it is made use of,
- and lastly, data is a non-rival good meaning that its use does not deplete its availability for future use or for others to use.
How companies measure information as an actual asset is a slippery concept. How do you mine unstructured data? What's the cost to produce the data? What is the potential future capital flows from the data? Are there economic and social impacts of data utilization? Consideration of potential strategic data values is essential before the implementation of costly data technologies.
Data classifications and repository architectures
Historically, progress in data technology was often associated with transistors or computational power like Moore’s law. According to industry experts, this nanometer law has reached its limit and the interest today is on the potential future of quantum computing.
Meanwhile, in recent years, the exponential growth of all sorts of data has shifted the focus toward data itself; such as data structures and data repositories. As a result, the complexity of “data” has progressed bringing forth not only technical but also societal and personal challenges.
In response to the technical challenges, big data architecture is geared to reply to business or organizational needs.
What is Big Data?
Big data is not one technology but an umbrella term used to describe the combination of a set of techniques, processing methods, and storage architectures with capabilities to manage a huge volume of mixed data at the right speed. Big data sets cannot be processed by traditional data management tools. To distinguish Big data from traditional, there are characteristics commonly referred to as 4Vs to indicate the size of data (Volume), the speed with which data is generated (Velocity), the types of data (Variety), and the quality of data (Veracity).
What makes data big important is variety since that is where data complexity and the need for advanced techniques arise. Basically, data variety is classified into three groups:
Structured, Semi-structured, and Unstructured
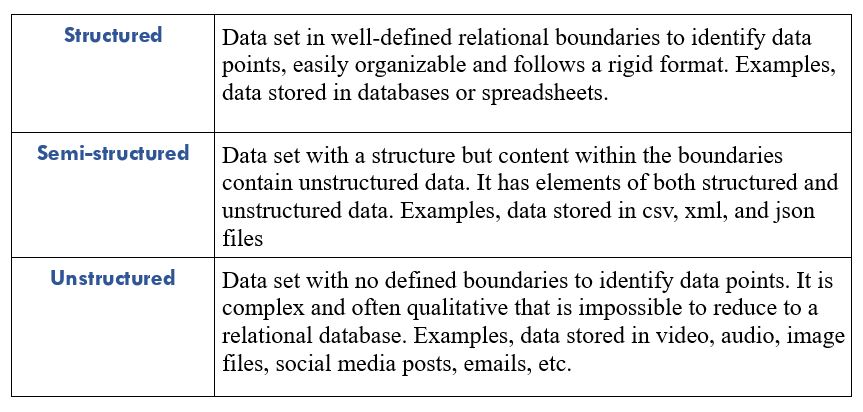
What articulates the goal of an enterprise strategy is to tap business value whether the data is structured or unstructured.
Historically, business data was mainly understood as part of the information hierarchy referred to as Data-Information-Knowledge (DIK).
The base structured data, collected from transaction processing systems (TPS), gets transformed into a relevant form to feed management information system (MIS) used by lower-level line managers. Further up in the hierarchy, information gets transformed into all sorts of reporting to supply decision support system (DSS) used by mid and higher management, and executive support system (ESS) used by top executives.
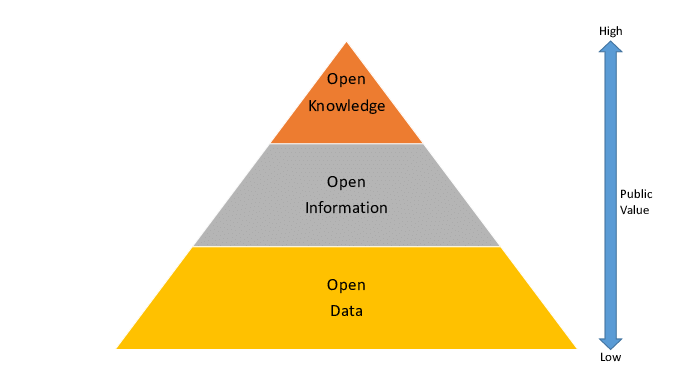
Data-Information-Knowledge hierarchy
Today, with growing data awareness and big data popularization, data has replaced information and knowledge in many contexts. Thus, many modern corporations have implanted data management centers (DMC) for their operations. Artificial intelligence (AI) algorithms help extract insights from the volumes of structured and unstructured data created daily. Business intelligence (BI) tools help the business make decisions
Data storage and management have evolved from simple databases to highly structured data warehouses, and in recent years, to much more flexible data lakes, to accomadate the growing need for unstructured data.
Although there are differences, it generally depends on the underlying practical application. Some companies engineer their data warehouses resembling data lakes while others choose strictly structured data warehouses. As technologies evolve, tech companies adopt the know-how of one another and differences start to disappear in time with market forces.
Data storage types
Costs and Value
Ultimately, the challenge remains on how to reduce operational costs and maximize data value.
I am no cost expert but experience has taught me that the more complex and structured a system is, the more it costs to operate. How people interact with data as a business strategy also determines cost variables. The higher the data users' literacy, the more agile the system and lower operational costs.
As to maximizing value, I think it all boils down to data cohesiveness and tech tools. Ending data silos for a scalable data hub is the first step in the value chain. Following that, the more data integration and interoperability occur, the more data value appreciates. And that logically leads to BI tools for business decision efficiency and to AI tools for learning from and adapting to new unstructured data sets.
Demand for “unstructured data” is increasing fast from multiple domains. In my next article, I will look at how unstructured data is used in specific contexts.
Further reading:
The Essentials of Market Data
Data Usage in Business
Sources:
Datacareer
Giplatform
Images:
Canva
Freepix
Wordart
Researchgate